Note
Click here to download the full example code
Seventh Example: Dynamically built component using initialization parametersΒΆ
- We continue demonstrating several interesting features:
- How the user can choose to encapsulate several blocks into a PipeGraph and use it as a single unit in another PipeGraph
- How these components can be dynamically built on runtime depending on initialization parameters
- How these components can be dynamically built on runtime depending on input signal values during fit
- Using GridSearchCV to explore the best combination of hyperparameters
import numpy as np
import pandas as pd
import matplotlib.pyplot as plt
from sklearn.linear_model import LinearRegression
from sklearn.preprocessing import MinMaxScaler
from sklearn.mixture import GaussianMixture
from pipegraph.base import PipeGraph, PipeGraph, Demultiplexer, Multiplexer, \
RegressorsWithParametrizedNumberOfReplicas
X_first = pd.Series(np.random.rand(100,))
y_first = pd.Series(4 * X_first + 0.5*np.random.randn(100,))
X_second = pd.Series(np.random.rand(100,) + 3)
y_second = pd.Series(-4 * X_second + 0.5*np.random.randn(100,))
X_third = pd.Series(np.random.rand(100,) + 6)
y_third = pd.Series(2 * X_third + 0.5*np.random.randn(100,))
X = pd.concat([X_first, X_second, X_third], axis=0).to_frame()
y = pd.concat([y_first, y_second, y_third], axis=0).to_frame()
We can think of programatically changing the number of models inside this component.
First we do it by using initialization parameters in a :class:PipeGraph
subclass
we called :class:pipegraph.standard_blocks.RegressorsWithParametrizedNumberOfReplicas
:
import inspect
print(inspect.getsource(RegressorsWithParametrizedNumberOfReplicas))
Out:
class RegressorsWithParametrizedNumberOfReplicas(PipeGraph, RegressorMixin):
def __init__(self, number_of_replicas=1, regressor=LinearRegression()):
self.number_of_replicas = number_of_replicas
self.regressor = regressor
steps = ([('demux', Demultiplexer())] +
[('regressor_' + str(i), clone(regressor)) for i in range(number_of_replicas)] +
[('mux', Multiplexer())]
)
connections = dict(demux={'X': 'X',
'y': 'y',
'selection': 'selection'})
for i in range(number_of_replicas):
connections['regressor_' + str(i)] = {'X': ('demux', 'X_' + str(i)),
'y': ('demux', 'y_' + str(i))}
connections['mux'] = {str(i): ('regressor_' + str(i)) for i in range(number_of_replicas)}
connections['mux']['selection'] = 'selection'
super().__init__(steps=steps, fit_connections=connections)
Using this new component we can build a simplified PipeGraph:
scaler = MinMaxScaler()
gaussian_mixture = GaussianMixture(n_components=3)
models = RegressorsWithParametrizedNumberOfReplicas(number_of_replicas=3, regressor=LinearRegression())
steps = [('scaler', scaler),
('classifier', gaussian_mixture),
('models', models), ]
connections = {'scaler': {'X': 'X'},
'classifier': {'X': 'scaler'},
'models': {'X': 'scaler',
'y': 'y',
'selection': 'classifier'},
}
pgraph = PipeGraph(steps=steps, fit_connections=connections)
pgraph.fit(X, y)
y_pred = pgraph.predict(X)
plt.scatter(X, y)
plt.scatter(X, y_pred)
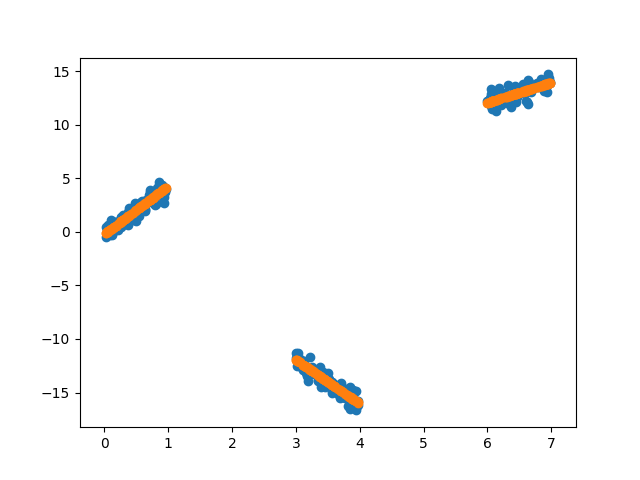
Total running time of the script: ( 0 minutes 0.037 seconds)