Note
Click here to download the full example code
Third Example: Injecting varying sample_weight
vectors to a linear regression model for GridSearchCV¶
This example illustrates a case in which a varying vector is injected to a linear regression model as sample_weight
in order to evaluate them and obtain the sample_weight that generates the best results.
Let’s imagine we have a sample_weight vector and different powers of the vector are needed to be evaluated. To perform such experiment, the following issues appear:
- The shape of the graph is not a linear sequence as those that can be implemented using Pipeline.
- More than two variables (typically:
X
andy
) need to be accordingly split in order to perform the cross validation with GridSearchCV, in this case:X
,y
andsample_weight
. - The information provided to the
sample_weight
parameter of the LinearRegression step varies on the different scenarios explored by GridSearchCV. In a GridSearchCV with Pipeline,sample_weight
can’t vary because it is treated as afit_param
instead of a variable.
Steps of the PipeGraph:
- selector: Featuring a
ColumnSelector
custom step. This is not a sklearn original object but a custom class that allows to split an array into columns. In this case,X
augmented data is column-wise divided as specified in a mapping dictionary. We previously created an augmentedX
in which all data buty
is concatenated and it will be used byGridSearchCV
to make the cross validation splits. selector step de-concatenates such data. - custom_power: Featuring a
CustomPower
custom class. A simple transformation of the input data that is powered to a specified power as indicated inparam_grid
. - scaler: implements
MinMaxScaler
class - polynomial_features: Contains a
PolynomialFeatures
object - linear_model: Contains a
LinearRegression
model
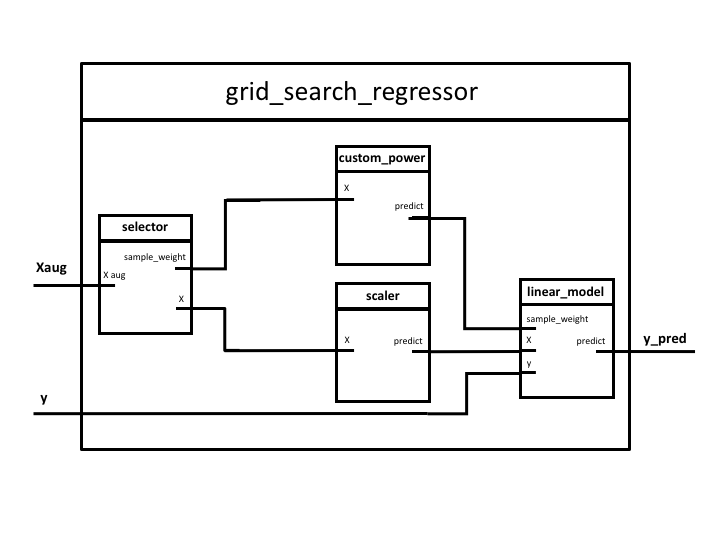
Figure 1. PipeGraph diagram showing the steps and their connections
import numpy as np
import pandas as pd
from sklearn.preprocessing import MinMaxScaler
from sklearn.preprocessing import PolynomialFeatures
from sklearn.linear_model import LinearRegression
from sklearn.model_selection import GridSearchCV
from pipegraph.base import PipeGraph, ColumnSelector, Reshape
from pipegraph.demo_blocks import CustomPower
import matplotlib.pyplot as plt
We create an augmented X
in which all data but y
is concatenated. In this case, we concatenate X
and sample_weight
vector.
X = pd.DataFrame(dict(X=np.array([ 1, 2, 3, 4, 5, 6, 7, 8, 9, 10, 11]),
sample_weight=np.array([0.01, 0.95, 0.10, 0.95, 0.95, 0.10, 0.10, 0.95, 0.95, 0.95, 0.01])))
y = np.array( [ 10, 4, 20, 16, 25 , -60, 85, 64, 81, 100, 150])
Next we define the steps and we use PipeGraphRegressor
as estimator for GridSearchCV
.
scaler = MinMaxScaler()
polynomial_features = PolynomialFeatures()
linear_model = LinearRegression()
custom_power = CustomPower()
selector = ColumnSelector(mapping={'X': slice(0, 1),
'sample_weight': slice(1,2)})
steps = [('selector', selector),
('custom_power', custom_power),
('scaler', scaler),
('polynomial_features', polynomial_features),
('linear_model', linear_model)]
pgraph = PipeGraph(steps=steps)
(pgraph.inject(sink='selector', sink_var='X', source='_External', source_var='X')
.inject('custom_power', 'X', 'selector', 'sample_weight')
.inject('scaler', 'X', 'selector', 'X')
.inject('polynomial_features', 'X', 'scaler')
.inject('linear_model', 'X', 'polynomial_features')
.inject('linear_model', 'y', source_var='y')
.inject('linear_model', 'sample_weight', 'custom_power'))
- Then we define
param_grid
as expected byGridSearchCV
exploring a few possibilities - of varying parameters.
param_grid = {'polynomial_features__degree': range(1, 3),
'linear_model__fit_intercept': [True, False],
'custom_power__power': [1, 5, 10, 20, 30]}
grid_search_regressor = GridSearchCV(estimator=pgraph, param_grid=param_grid, refit=True)
grid_search_regressor.fit(X, y)
y_pred = grid_search_regressor.predict(X)
plt.scatter(X.loc[:,'X'], y)
plt.scatter(X.loc[:,'X'], y_pred)
plt.show()
power = grid_search_regressor.best_estimator_.get_params()['custom_power']
print('Power that obtains the best results in the linear model: \n {}'.format(power))
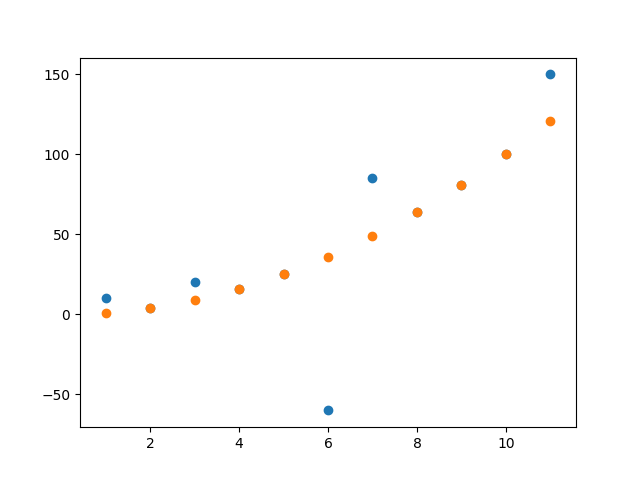
Out:
Power that obtains the best results in the linear model:
CustomPower(power=20)
This example displayed a non linear workflow successfully implemented by PipeGraph, while at the same time showing a way to circumvent current limitations of standard GridSearchCV
, in particular, the retriction on the number of input parameters.
Next examples show more elaborated examples in increasing complexity order.
Total running time of the script: ( 0 minutes 0.519 seconds)